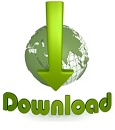
Although the language modeling approach has performed well empirically, a significant amount of performance increase is often due to feedback. The relative simplicity and effectiveness of the language modeling approach, together with the fact that it leverages statistical methods that have been developed in speech recognition and other areas, make it an attractive framework in which to develop new text retrieval method. The language modeling approach to text retrieval was first introduced by Ponte and Croft in and later explored in –. As a result, improving the effectiveness of search results for such difficult queries would bring user satisfaction which is the ultimate goal of search engines. In such a case, a user would have to either reformulate the query or go far down on the ranked list to examine more documents. Indeed, a query might be so simple or ambiguous that a large number of top-ranked documents are non-relevant, and we usually call it difficult query.
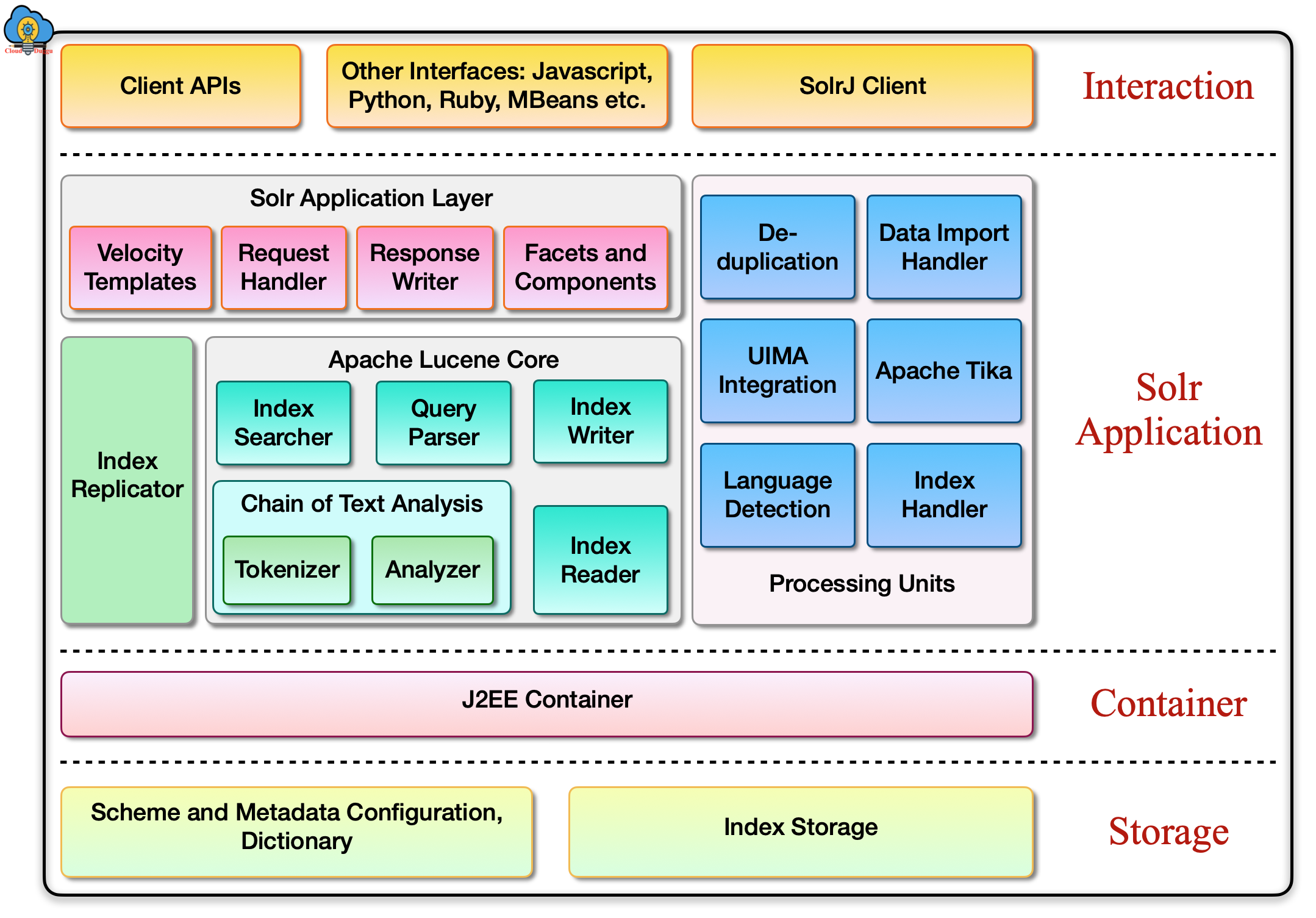
Since the inherent limitations of current retrieval models, it is nearly impossible for any retrieval model to return satisfactory results for every query. The funders had no role in study design, data collection and analysis, decision to publish, or preparation of the manuscript.Ĭompeting interests: The authors have declared that no competing interests exist. 08BTQ025), the Project Sponsored by the Scientific Research Foundation for the Returned Overseas Chinese Scholars, State Education Ministry and The Research Fund for the Doctoral Program of Higher Education (No. 2006AA01Z151), National Social Science Foundation of China (No. 6067303068), the National High Tech Research and Development Plan of China (No. This is an open-access article distributed under the terms of the Creative Commons Attribution License, which permits unrestricted use, distribution, and reproduction in any medium, provided the original author and source are credited.įunding: This work is supported by grants from the Natural Science Foundation of China (No. Received: MaAccepted: JPublished: August 19, 2014Ĭopyright: © 2014 Ma, Lin. PLoS ONE 9(8):Įditor: Rongrong Ji, Xiamen University, China Experimental results on these TREC collections show that the proposed language model based multiple model feedback method which is generally more effective than both the baseline method and the methods using only positive or negative model.Ĭitation: Ma Y, Lin H (2014) A Multiple Relevance Feedback Strategy with Positive and Negative Models. Then, we conduct a novel study of multiple strategies for relevance feedback using both positive and negative examples from the first-pass retrieval to improve retrieval accuracy for such difficult queries.
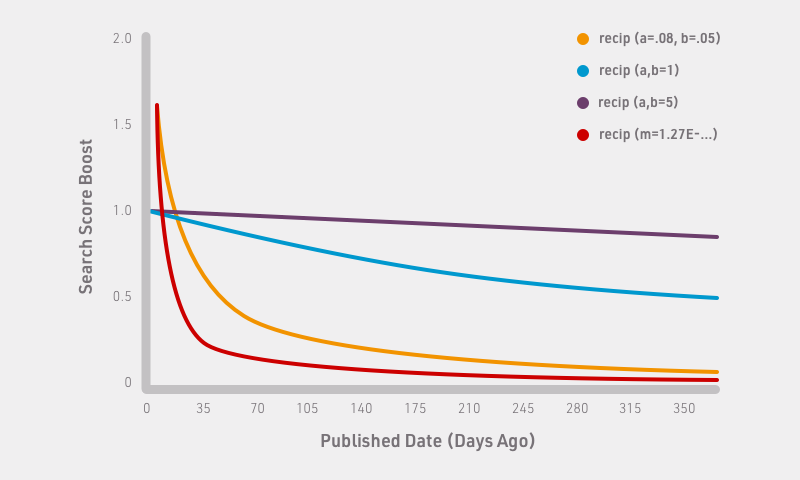
In this paper, we consider a scenario when the search results are so poor that there are at most three relevant documents in the top twenty documents. Contrarily, there is a relatively large number of negative documents in the top of the result list, and it has been confirmed that negative feedback strategy is an important and useful way for adapting this scenario by several recent studies. Therefore, the positive feedback strategy is incapable to improve retrieval in this situation. However, when a query topic is difficult and the results from the first-pass retrieval are very poor, it is impossible to extract enough useful terms from a few positive documents. Most existing work on feedback relies on positive information, and has been extensively studied in information retrieval. A commonly used strategy to improve search accuracy is through feedback techniques.
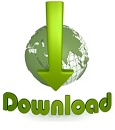